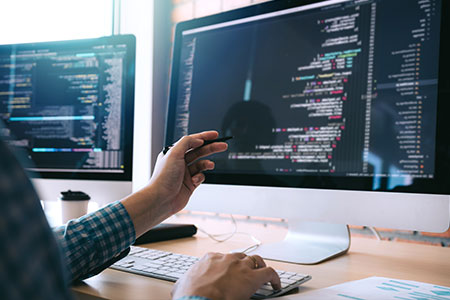
The need for constant data quality management
In order to take full advantage of the massive amount of data that is accumulated every day, the quality of the data must be excellent.
First, data quality can be secured only when data quality management system is established by defining data standards and rules, and continuous data quality monitoring and improvement work is performed based on this.